Machine Learning Model for Superconductor Critical Temperature
Based on Graph Neural Networks
Computation may take up to 10 seconds, due to network limit. This model is currently deployed on Microsoft Azure and the Research Computing facilities at ASU.
Cite this model:
Si-Da Xue and Qi-Jun Hong, Materials Properties Prediction (MAPP): Empowering the prediction of material properties solely based on chemical formulas, arXiv, 2023. Download.
Metrics
R2 training score: 0.92
R2 testing score: 0.90
Root mean square error, training: 8.5K
Root mean square error, testing: 9.0K
About this model
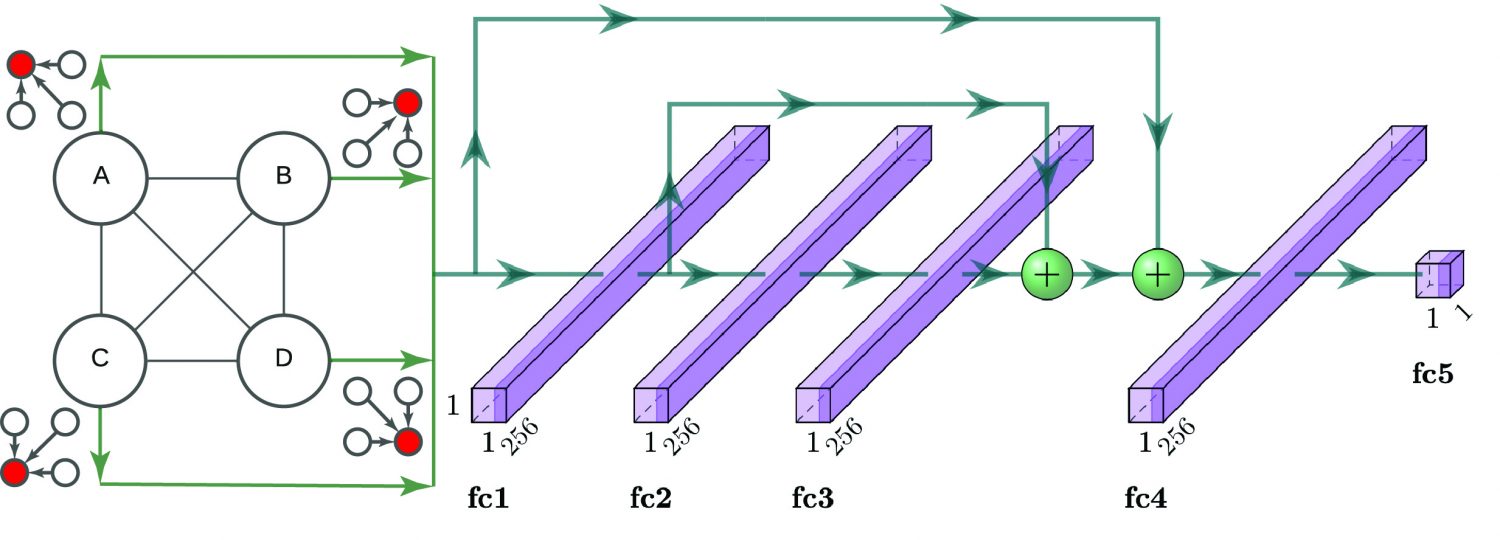
Video Introduction
Here is a clip from my talk at the TMS 2022 conference.